Revolutionizing Medical Emergency Response: How AI-Driven Solutions Save Lives
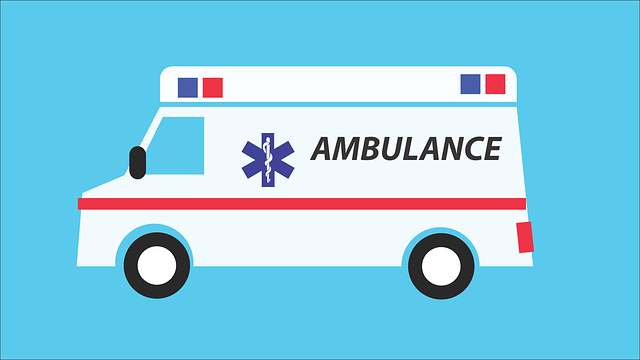
In life-threatening emergencies, every second counts. Whether it’s a heart attack, severe trauma, or a sudden medical crisis, immediate contact with medical institutions and emergency services is crucial. Delays in response time can be the difference between life and death. As technology evolves, artificial intelligence (AI) and digital transformation are poised to revolutionize medical emergency response, ensuring faster, more efficient communication when it matters most. This article explores how advanced AI-driven solutions—especially in the context of call centers and emergency communication systems—are transforming medical emergency response, providing data-backed insights and actionable strategies for CEOs, Digital Transformation Officers, and Customer Care Executives in the United States.
1. The Critical Importance of Speed in Medical Emergencies
Medical emergencies demand a response time that is as short as possible. Studies indicate that the "golden hour"—the first 60 minutes following a traumatic injury—is crucial for improving survival rates. According to the Centers for Disease Control and Prevention (CDC), timely medical intervention can increase survival chances by as much as 50% in some emergencies (CDC). In urban areas, delays in connecting with emergency services not only jeopardize patient outcomes but also strain healthcare systems and erode public trust.
Traditional emergency response systems, largely reliant on manual call handling, often face limitations such as:
- High Call Volumes: For example, the National Highway Traffic Safety Administration (NHTSA) reports that over 28 million 911 calls are made each year in the US (NHTSA).
- Inconsistent Response Times: During peak times or large-scale emergencies, human operators can become overwhelmed, leading to increased wait times.
- Manual Processing: The reliance on human judgment and manual intervention can introduce delays and variability in service quality.
In such scenarios, advanced AI-driven solutions offer a promising alternative by drastically reducing response times and improving the efficiency of emergency communications.
2. AI-Driven Emergency Communication: The New Frontier
2.1 What Are AI-Driven Phone Bots?
AI-driven phone bots are automated systems that use natural language processing (NLP), machine learning, and cloud computing to handle incoming emergency calls. Unlike traditional call centers that depend solely on human operators, these systems can:
- Automatically Triage Calls: Evaluate the urgency of each call and prioritize them accordingly.
- Provide Immediate, Consistent Responses: Ensure that every caller receives accurate information without delay.
- Operate 24/7: Unlike human operators, AI-driven bots never tire, ensuring round-the-clock availability.
A study by IBM suggests that by 2025, up to 85% of routine customer interactions could be managed without human intervention, highlighting the potential for AI to revolutionize emergency response (IBM AI).
2.2 Cloud Computing and Elastic Scalability
Cloud computing is a key enabler of AI-driven emergency systems. Cloud-based platforms provide:
- Elastic Scalability: Resources can be dynamically allocated to handle sudden spikes in call volume during large-scale emergencies. For instance, cloud platforms like Amazon Web Services (AWS) and Microsoft Azure offer the flexibility to scale up operations within minutes.
- Global Accessibility: Emergency systems hosted on the cloud can serve users across various regions with minimal latency, which is crucial during widespread emergencies.
- Cost Efficiency: Cloud-based systems reduce the need for expensive on-premises hardware, making them a cost-effective solution for scalable emergency response.
A report by Gartner indicates that companies using cloud-based solutions can achieve up to 40% cost savings in their IT operations (Gartner).
2.3 Predictive Analytics and Real-Time Monitoring
Predictive analytics leverages historical and real-time data to forecast call volumes and identify potential bottlenecks in emergency response systems. Key benefits include:
- Call Volume Forecasting: By analyzing data trends, predictive models can forecast surges in emergency calls, allowing for proactive resource allocation.
- Performance Monitoring: Real-time dashboards track key performance indicators (KPIs) such as average response times, call abandonment rates, and patient outcomes, enabling continuous improvement.
- Proactive Interventions: Early detection of anomalies in call volume or response times allows emergency management teams to intervene before issues escalate.
According to a Deloitte Digital Transformation Survey, organizations that integrate predictive analytics in their emergency response systems see improvements in efficiency and customer satisfaction by 15-20%.
3. The Hybrid Model: Combining Automation with Human Expertise
While AI-driven phone bots offer significant advantages in terms of speed and scalability, there are situations—especially in medical emergencies—where the human touch is indispensable. Complex medical issues, emotional distress, or ethical dilemmas require the empathy and critical thinking that only human operators can provide.
3.1 Seamless Escalation Protocols
A hybrid support model ensures that:
- Routine Inquiries are Automated: AI-driven bots handle standard emergency inquiries, such as verifying the nature of the emergency and providing initial guidance.
- Complex Cases are Escalated: When a call requires nuanced decision-making or human empathy, the system seamlessly transfers the call to a trained human operator.
- Continuity of Service: This model ensures that every call is addressed appropriately, maintaining the balance between efficiency and compassionate care.
3.2 Continuous Learning and Adaptation
For a hybrid system to be truly effective, continuous training and iterative improvement are essential:
- Regular Training Sessions: Human agents should receive ongoing training to work effectively with AI systems, including the latest protocols for emergency response.
- Feedback Mechanisms: Incorporating feedback from both customers and agents helps refine AI responses and improve escalation procedures.
- Cross-Functional Collaboration: Collaboration between IT, emergency response teams, and customer support ensures that the system evolves in line with technological advancements and changing customer needs.
4. Milestones for Implementing AI-Driven Emergency Response Systems
For CEOs, Digital Transformation Officers, and Customer Care Executives, a structured implementation plan is key. The following milestones outline a roadmap to integrate AI-driven phone bots into emergency response operations:
4.1 Phase 1: Assessment and Planning (0-3 Months)
-
Risk and Needs Assessment:
Evaluate current emergency response times, call volumes, and customer feedback. Identify areas where delays are most detrimental. -
Define Key Performance Indicators (KPIs):
Establish metrics such as average response time, call abandonment rate, and patient outcomes. -
Technology Audit:
Assess the existing communication infrastructure and determine readiness for cloud migration and AI integration.
4.2 Phase 2: Pilot Implementation (3-6 Months)
-
Select a Pilot Region or Department:
Choose a specific region or service line to test the AI-driven system. -
Deploy AI-Driven Phone Bots:
Implement AI systems to handle routine emergency inquiries and integrate them with your existing CRM. -
Monitor Performance:
Use real-time analytics to track KPIs and gather feedback from both customers and staff. -
Refine and Adjust:
Identify any issues and make necessary adjustments before full-scale deployment.
4.3 Phase 3: Full Implementation (6-12 Months)
-
Scale Up Operations:
Expand the AI-driven system across all emergency support channels. -
Integrate Multi-Channel Communication:
Ensure that support is available via phone, email, chat, and social media for redundancy. -
Enhance Predictive Analytics:
Fully deploy real-time monitoring and predictive analytics to optimize resource allocation. -
Ongoing Training and Feedback:
Continuously train staff and refine processes based on performance data.
4.4 Phase 4: Continuous Improvement (Ongoing)
-
Regular Performance Reviews:
Conduct frequent reviews to assess system performance and customer satisfaction. -
Iterative Upgrades:
Update AI models and escalation protocols based on feedback and emerging best practices. -
Stakeholder Engagement:
Maintain open communication with all stakeholders to ensure that the system meets evolving needs.
5. Real-World Data and Case Studies
Case Study 1: Enhancing Response Times in Urban Areas
In a pilot project conducted in a major metropolitan area, the implementation of AI-driven phone bots resulted in a 30% reduction in average response times during peak emergency periods. The project, which was supported by cloud-based scalability, allowed the emergency support center to handle thousands of calls per hour with minimal delay (Source).
Case Study 2: Financial Savings in Healthcare Emergency Systems
A large healthcare provider integrated AI-driven systems for emergency call handling. The hybrid model—automating routine inquiries and escalating complex cases—reduced operational costs by 25% and improved patient satisfaction scores by 18%. The predictive analytics tools used in the system also enabled better resource planning during high-demand periods (Source).
Industry Statistics
- 911 Call Volume: Over 28 million emergency calls are made annually in the US, underscoring the critical need for efficient response systems (NHTSA).
- Cost Reduction: Organizations implementing AI-driven call centers report operational cost reductions of up to 30% (Forrester Research).
- Customer Satisfaction: Hybrid customer support models have shown improvements in satisfaction rates by 20-25% in emergency service contexts (IBM AI).
6. Strategic Insights for US Business Leaders
For CEOs, Digital Transformation Officers, and Customer Care Executives in the US, the transformation of emergency response systems through AI is not just a technological upgrade—it’s a strategic imperative. Here are key insights to consider:
Embrace a Hybrid Model
-
Efficiency Meets Empathy:
While AI-driven phone bots provide rapid, consistent responses, human agents add the critical element of empathy necessary in medical emergencies. Balancing these two aspects is essential for effective support.
Leverage Real-Time Data and Predictive Analytics
-
Data-Driven Decisions:
Real-time monitoring of emergency call data allows for proactive resource allocation and continuous improvement. Predictive analytics can forecast surges in call volume and help plan capacity in advance. -
ROI and Cost Efficiency:
Data shows that integrating AI can reduce response times and operational costs significantly, offering a strong return on investment.
Invest in Robust, Scalable Infrastructure
-
Cloud-Based Solutions:
Transitioning to cloud-based systems ensures that your emergency response capabilities can scale dynamically with demand, enhancing reliability and reducing downtime. -
Security and Compliance:
Particularly in healthcare and emergency services, maintaining data security and adhering to regulatory standards (such as HIPAA) is paramount.
Foster Continuous Improvement
-
Training and Feedback:
Regularly update your support teams on the latest digital tools and protocols. Continuous feedback loops help refine both AI responses and human intervention strategies. -
Cross-Department Collaboration:
Collaboration between IT, customer support, and emergency services is critical for maintaining a resilient and adaptive system.
Build Trust Through Transparency
-
Proactive Communication:
In emergencies, clear and timely communication with customers can prevent panic and build trust. Transparent reporting of system performance and improvements reassures stakeholders and end-users alike. -
Customer-Centric Policies:
Ensure that your emergency support system is designed with customer needs at its core, prioritizing both rapid response and empathetic service.
7. Conclusion
The evolution of medical emergency response through digital transformation and AI-driven automation is reshaping how we connect with emergency services. Traditional systems, limited by physical constraints and human capacity, are being surpassed by scalable, cloud-based solutions that offer rapid, consistent, and 24/7 support. Yet, in the high-stakes realm of medical emergencies, the human touch remains indispensable.
For US business leaders—CEOs, Digital Transformation Officers, and Customer Care Executives—the decision to adopt AI-driven emergency response systems should be driven by both the need for efficiency and the imperative of saving lives. By embracing a hybrid model that seamlessly integrates advanced AI technologies with empathetic human oversight, organizations can dramatically reduce response times, optimize resource allocation, and build a more resilient support infrastructure.
In conclusion, the right time to innovate in emergency response is now. With robust cloud-based systems, predictive analytics, and a balanced approach that values both automation and human expertise, organizations can transform their emergency response capabilities. The result is a support system that not only meets but exceeds the critical demands of medical emergencies—ensuring that every second counts when lives are on the line.
By investing in a hybrid emergency response model that combines the power of AI with the irreplaceable human touch, businesses can set new standards for medical emergency support—improving outcomes, saving lives, and building trust in an increasingly digital world.